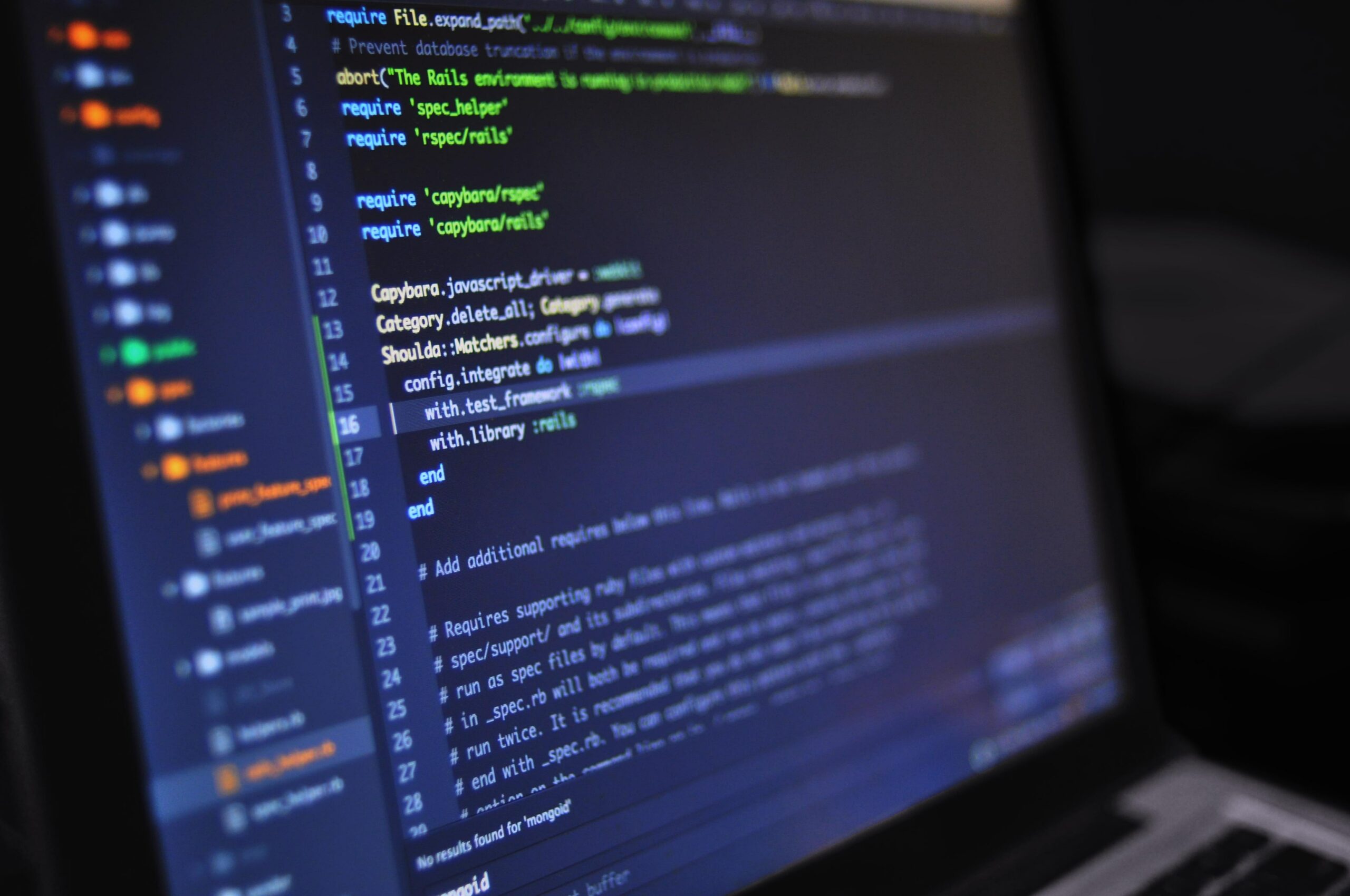
Addressing Algorithmic Discrimination in the European Union
24 November, 2020
On February 5, 2020, the District Court of the Hague ruled that SyRI, or Systeem Risico Indicatie (System Risk Indicator), could no longer be used in low-income Dutch neighborhoods to flag individuals more likely to commit benefits fraud. First implemented in 2014 by the Dutch Ministry of Social Affairs, the program collected 17 categories of government data (tax records, land registry files, vehicle registrations) from residents living in low-income and immigrant neighborhoods in Rotterdam, Eindhoven, Capelle aan den Ijssel, and Haarlem. It then ran this information through a predictive algorithm and assigned values to each household indicating the level of risk to benefits agencies. The court determined not only that the General Data Protection Regulation (GDPR) prohibits the collection of personal data on this scale, but that by predetermining anyone living in the wrong area as more likely to commit a crime, SyRI constitutes a human rights violation.
According to Christian van Veen, director of the Digital Welfare State and Human Rights Project at New York University, the SyRI ruling might influence how other courts and countries interpret EU human rights law and privacy law. Its language has far-reaching implications for how human rights and privacy law is applied to predictive algorithms in a policy context. The Hague determined SyRI was a violation of the European Convention of Human Rights and GDPR, even though the Dutch government stressed that it was neither used to trigger a full-fledged investigation on nor legal consequences for those who might have been flagged as high-risk. However, the content of the stored data itself, the intent behind collecting this data, and the inherent power asymmetry between the Dutch government and the low-income urban inhabitants who were being surveilled en masse was sufficient enough to prompt a negative court ruling—regardless of its actual use.
The SyRI ruling is highly illustrative of how artificial intelligence could be potentially misused for discriminatory purposes. Rather than technology becoming an equalizer in society, it can be used to simply reinforce pre-existing power imbalances by concentrating and centralizing information into the hands of powerful authorities and individuals who have the financial and technical means to analyze the data and utilize it towards their own ends.
Artificial intelligence in itself is inherently blind to any context outside of the data that is fed into it, and it therefore has the potential to draw faulty conclusions from the viewpoint of its human observers. What the algorithm determines to be the correct approach to solving a human problem, when taken in the context of society, has the potential to be discriminatory. Constant risk assessments throughout the lifecycle of an AI project with multiple stakeholders, which must also include ethical impact assessments, is the preferred recommendation by multiple AI experts to help address the problem.
Is AI really intelligent?
The most well-known test for deciding if a machine is “artificially intelligent” is the Turing Test. Under its standard interpretation, two players, A and B, where A is a machine, exchange written notes with an interrogator C, who cannot see them. A has to pretend to be a human and convince the interrogator that it is one. If the interrogator guesses wrongly that A is a human multiple times, then by the criteria of the Turing test, A would be considered a thinking computer.
The fact is, however, that the one who is deciding whether the computer is “intelligent” or not is, at the end of the day, a fallible human. The human interrogator may have preconceived ideas of how a machine or a human might act which do not match reality. The one who is deciding on the correctness of the machine’s approach may certainly have biases of their own that influence the development of the machine’s thinking capabilities through human feedback.
In addition to the human biases present in the human beings training the machines, there is also the fact that algorithms themselves exhibit bias. A recent American study published in Science examined a widely used commercial prediction algorithm employed to identify patients who need additional health interventions, and found that the algorithm ranked white patients at similar levels of risk as black patients—even though black patients exhibit greater uncontrolled illnesses than whites. If this racial disparity were corrected, stated the authors, then the percentage of black patients in the United States receiving additional help would increase from 17.7% to 46.5%.
As reported by the study’s authors, the cause of this bias is the use of healthcare costs as a predictor of healthcare risk. Since white patients in the United States have greater access to healthcare than black patients, the amount of money spent on their care is greater, which the algorithm interprets as a greater risk indicator of disease. The use of simple, convenient proxies for ground truth, note the authors, can be a source of considerable algorithmic bias, as can the economic and cultural context in which the algorithm was developed.
Legal Implications
Since, by definition, machine learning algorithms run automatic correlations on various sets of data with the aim of extrapolating general trends, the application of non-discrimination laws to the outcomes of artificial intelligence must be clearly articulated. According to Raphaële Xenidis and Linda Senden, algorithmic bias and algorithmic discrimination do not map evenly onto each other from the perspective of European human rights law, and the realities of the adoption of this technology runs up against the robustness of the European legal and regulatory framework in a number of ways.
The way algorithms draw discriminatory conclusions is often not overt, but rather derives from how systemically-encoded disadvantages could show up in the data itself. To take the example of an algorithm meant to predict the likelihood of promotion within the workplace, we assume the data used to “train” the model is made up of past successful promotions. Because women are more likely to work less hours or take parental time off, the algorithm may conclude that being male makes you more likely to be promoted. Even if it is made blind to gender as a variable, the model may still exhibit bias towards male employees because “working hours,” in this instance, have become a proxy for gender.
Philipp Hacker reduces the sources of algorithmic discrimination to two main points. The first is “biased training data,” in which, as in the above example, data is skewed because of historical discrimination or the answer taught to the algorithm is false. The second, “unequal ground truth,” refers to the fact that variables consisting of unprotected categories, such as working hours or zip code, may become indicators that point to protected categories, such as gender or race.
The EU forbids feeding personally-identifying data into machine learning algorithms without the explicit consent of the user through the GDPR. Furthermore, uses of personally-identifying information that lead to discriminatory outcomes fall explicitly into the definition of direct discrimination. However, regulators are becoming more aware that structural and social imbalances are multifaceted, and the aforementioned fact that non-protected data may serve as proxies for protected data raises a large number of issues that need to be addressed.
A definition of “indirect discrimination” appears in both Article 2(2)(b) of Directive 2000/43/EC and Article 2(1)(b) of Directive 2006/54/EC, “…where an apparently neutral provision, criterion or practice would put [members of a protected category] at a particular disadvantage compared with other persons, unless that provision, criterion or practice is objectively justified by a legitimate aim and the means of achieving that aim are appropriate and necessary.” This could easily apply to instances of structurally discriminatory data encoding or unequal ground truth insofar as they affect the end user in a tangible way.
According to Christopher McCrudden and Sacha Prechal, when practices that “formally apply to all” have the “effect of disadvantaging individuals of protected groups,” then antidiscrimination law applies. However, unless those practices can be shown to have a “legitimate aim” and the means of achieving that aim are “appropriate and necessary,” Xenidis and Senden point out that the courts are unlikely to accept blanket justifications for the use of “unfair” or “biased” algorithms except in narrowly-tailored instances.
What measures should be taken?
Algorithmic discrimination is a highly multifaceted problem which will require action and training on the part of machine learning professionals, business leaders, legal experts, and policymakers. These groups will need to work in tandem to reach a common outcome. Per the recommendations of many prominent members of the European AI community, the regulatory framework across the member states must be harmonized at an intergovernmental and supranational level, namely via a common set of “Trustworthy AI” standards. Such measures include setting up a separate regulatory body to define and ensure compliance with “Trustworthy AI” standards, regular audits at each stage of the production process, and fostering the creation of “trusted data spaces” for specific sectors.
One must take into account that the feasibility of complying with these measures rests primarily on the level of subject-matter expertise held by the AI engineers themselves regarding the type of data that they are working with. The identification of faulty cross-correlations and structural inequalities embedded in the data would have to take place during the so-called “feature engineering” stage of the machine learning lifecycle, whereby class imbalances (i.e., an overrepresentation of male subjects) and redundant variables which correlate closely with each other (such as a correlation between healthcare spending and race) are rectified before being fed into the machine learning algorithm.
Furthermore, how complex “hyperparameters” are tuned within the algorithm will determine whether the algorithm is simply “memorizing” the data or whether it is actually able to extrapolate trends and predict outcomes in the future. The testing cycle for verifying results is already iterative and fluid because of the complexity of the variables used. Therefore, ensuring compliance with antidiscrimination law would place a greater onus on private industry and, ultimately, require closer collaboration between the technological and regulatory areas of companies.
The last important issue which needs to be further articulated is whether the prohibition on collecting personal demographic data under the GDPR means that AI professionals must ensure compliance with non-discrimination rules by extrapolating the results from indirect variables. Put simply, one may argue that including information like race and gender in a machine learning model could help professionals more easily identify and correct demographic imbalances in the results.
At the end of the day, the need for a balanced approach to antidiscrimination law in the context of AI will not only need to rely on the experts, but also the testimonies of those affected. The case of SyRI, for example, showed how a “blind” machine learning algorithm was brought down by the testimonies of affected community members themselves—who were, ultimately, the ones who shone a light into the algorithmic black box.